Advance Australia FAIR
WESLEY LAWRENCE, 2021
Recently returned from the National Farmers Federation (NFF) Towards 2030 forum I have had the pleasure of having some highly engaging conversations with the good people of the National Farmers Federation (NFF), WA Farmers Federation (WAFarmers) along with government, growers and industry around technology and data and the role this is, and will continue to, play in agriculture.
- Customers & the Value Chain
- Growing Sustainability
- Unlocking Innovation
- People & Communities
- Capital & Risk
It is clear that technology and data will have a very significant role to play in meeting these aims, not just within the Unlocking Innovation pillar, but throughout. Some shapes and forms this is taking and may continue to take are:
- Customer trust being supported and grown through producer claims on clean, green, chemical use, animal welfare and sustainability which are underpinned, evidenced and communicated by technology and data
- Value chains being interconnected through data and technology
- Sustainability being measured, evidenced, certified and improved upon through data and technology
- Unlocking emerging technologies like IoT, machine learning, robotics and automation generating and consuming vast qualities of data
- Data driven analysis, compliance, investment, management and insurance.

The FAIR data principles of Findable, Accessible, Interoperable, and Reusable (FAIR) have been adopted by Australian National Data Service (ANDS), Australian Research Data Commons (ARDC) and CSIRO among others with the aim to “support knowledge discovery and innovation both by humans and machines, support data and knowledge integration, promote sharing and reuse of data, be applied across multiple disciplines and help data and metadata to be ‘machine readable’, support new discoveries through the harvest and analysis of multiple datasets and outputs.” (ARDC website, https://ardc.edu.au/resources/working-with-data/fair-data/, 2021)
FAIR data principles have applicability across the breadth of agriculture and agribusiness, with applicability across any commodity type and production system regardless of size or scale, encompassing farms, agribusinesses, food and beverage processors, grower groups, consultants, RDC’s and government, academic and industry research. The principles apply internally for a business or organisation, and guide considerations for data ingestion and standardisation, data storage, on-farm data consumption and external or aggregated data consumption such as research collaborations, data sharing, data hubs and aggregated consumption platforms like AgReFed. Pillars 5-8 for those familiar with the 8 Pillars of AgTech.
It is vitally important to clarify that the FAIR data principles are not a framework that constitutes free or freely shared data, nor are they levers for coercing farmers or businesses into sharing data as if they are the sibling that has a packet of lollies and mum has told them they have to share. The principles are about evolving and guiding capability, capacity and preparedness and ensuring there is sufficient controls to allow for active and willing consent.
Findable
For data that is still in paper form or electronic documents (#farmrecordsaredatatoo) findable may take the form of a catalogue that outlines what data exists where. An audit or mapping exercise is frequently used as a tool for industrial businesses in understanding where their data is and what shape and format it is in. For data that is already in digital and data form, well organised and populated metadata, or data about the data, becomes crucial to creating findable data.
Accessible
Accessible isn’t about data being free and freely downloadable, it is partly about the shape and form it is in. A bunch of PDFs on a thumb drive or a filing cabinet full of historical records is not very accessible. The other part of accessible is about a mechanism by which data can be accessed. This may be layer of trust and consent – access in a particular way, for a particular purpose, for a particular period of time, for a particular value exchange (quid-pro-quo, something for something).
Interoperable
In this current burst of technology and platforms it is highly unlikely that any one provider or one platform will universally win the day. In some arenas or in some production areas or in some markets perhaps, but data is so widely usable that there will always be someone sitting outside of any given platform. It is far more likely that the technology market will evolve to being a series of interconnected platforms where data flows from one to another according to its use case. In this scenario interoperability and API’s (Application Programming Interfaces that allow data to be served from one software to another) will be the order of the day. To facilitate this, data will need to become more aligned through the use of community accepted formats, vocabularies identifiers and units in the data and metadata.
Reusable
Reuse of data is where the rubber hits the road in terms of value. The quality of that data directly impacts on its ability and readiness for reuse. Pat Kennedy, the developer of an industrial operational data framework used by industry globally, held the mantra that “Data is one of the few things that becomes more valuable the more times it is used”. Data reuse can and will take many different shapes and forms – internal analysis and benchmarking, external analysis and benchmarking, certifications and compliance, machine learning, automation to name a few, plus ways that haven’t even yet been imagined. Reusability is facilitated by well organised and well structured data with rich contextual metadata.
The work required in making data FAIR is about both making it easier to generate ROI’s from data and creating a data legacy. For the producer looking at a succession plan, there is the question of the shape and form of that data legacy and its readiness for what the future may bring. For the government, academic, industry, grower or grower group researcher it is about the shape and form of the data after the paper is published or the project is finished and its usability for those that come next.
By way of update on the NFF 2030 target, the industry is sitting at $61b for 2019-2020 FY and is forecast to hit $84b by 2030 at current trajectory with a $16b gap. In terms of alignment with the guiding principles, lots are progressing but there are shortfalls, some are about messaging and some require real work. Perhaps making data fair and FAIR can help on both counts.
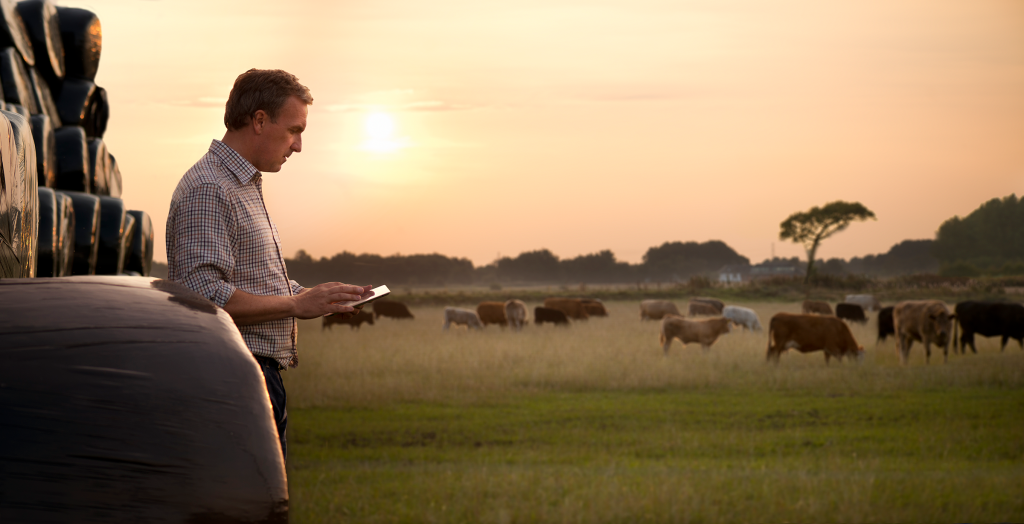